Adopting Gen AI & ML in Full Stack Observability for ITOps
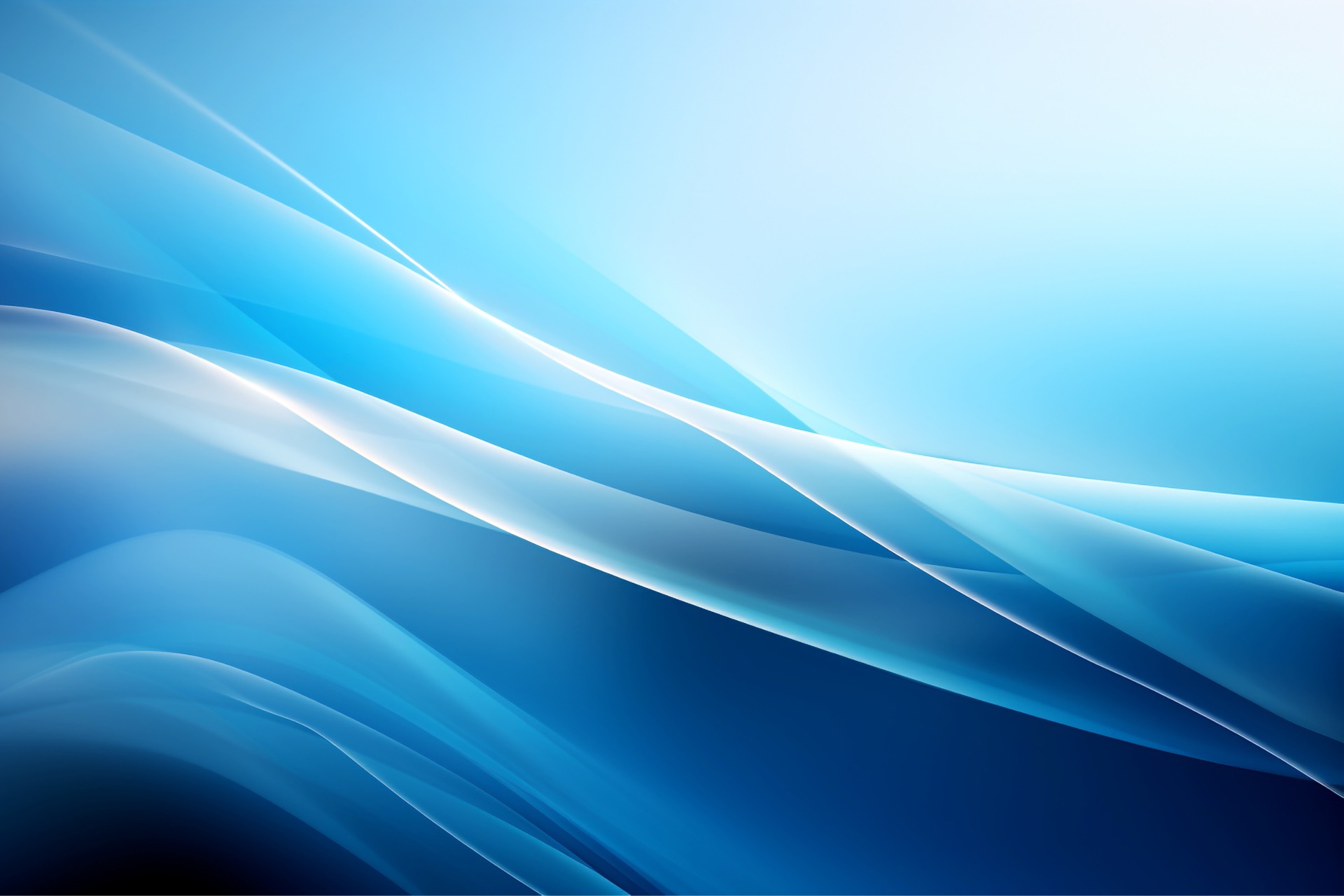
In July 2024, Delta Air Lines faced a significant operational disruption due to a global IT outage linked to a faulty software update from cybersecurity firm CrowdStrike. This incident led to the cancellation of over 7,000 flights, affected more than 1.3 million passengers, and caused substantial financial and reputational damage to the airline1. Incidents like these highlight a growing reality: our digital systems are deeply intertwined with real-world outcomes. When something breaks, it’s rarely due to a single failure. It’s often the result of multiple issues hiding deep within the tech stack.
This is where Full-Stack Observability, powered by Gen AI and Machine Learning, becomes critical. It offers a comprehensive approach to understanding system behaviors across the entire technology stack. It enables organizations to proactively identify anomalies, predict potential failures, and implement automated responses, thereby minimizing downtime and ensuring seamless operations.
According to a 2024 Gartner report, by 2025, 70% of digital businesses will require infrastructure observability as a core capability, up from just 25% in 20222. At Tech Mahindra, we enable organizations to stay ahead of the curve, equipping them with Gen AI-driven observability that delivers performance, reliability, and strategic insights.
Key Benefits of applying Gen AI-based Observability in our operations
At its core, Full-Stack Observability aggregates telemetry data—logs, metrics, events, and traces—across the stack, enabling end-to-end visibility and proactive issue resolution. When coupled with Gen AI and ML, observability platforms evolve from reactive dashboards into intelligent, predictive engines. Here are a few key benefits:
- Faster Problem Resolution: AI-driven anomaly detection and RCA (Root Cause Analysis) accelerate MTTD and MTTR through autonomous diagnostics.
- Improved Performance: Continuous performance profiling helps pinpoint bottlenecks across services, APIs, databases, and various layers of infrastructure.
- Enhanced User Experience: Real User Monitoring (RUM) and Synthetic Monitoring provide a granular view of UX across geographies and devices.
- Optimized Resource Utilization: ML algorithms adjust compute, memory, and network allocations dynamically, ensuring cost efficiency.
- Predictive Analysis: LLMs forecast workload spikes and performance degradations, enabling preemptive action before users are impacted.
Evolution of the Platform: From Reactive Monitoring to Gen AI-Enabled Full-Stack Observability
The evolution of monitoring platforms reflects the growing complexity and expectations of enterprise IT environments. From simple ping-based uptime checks to AI-powered analytics, observability has undergone a significant transformation.
- Before the 2000s: Monitoring was largely reactive, relying on installed agents to track basic device availability using protocols such as Ping, Telnet, and Netstat. Monitoring was confined to IP-based infrastructure and focused solely on uptime.
- Early 2000s: The emergence of agentless monitoring using SNMP and Syslog laid the groundwork for scalable network monitoring. Tools focused on infrastructure metrics (CPU, memory, network I/O) and introduced graphical dashboards for centralized visibility.
- Late 2000s–2010s: With the advent of virtualization and cloud computing, monitoring tools expanded to support hybrid environments. They enabled both agent-based and agentless tracking through WMI, SSH, and APIs. Basic application monitoring features were added, along with ITSM integrations for automated incident generation.
- Mid-2010s: As microservices, containers, and distributed systems became the norm, observability platforms incorporated advanced features such as Remote PowerShell, SNMP Traps, and API integrations. Dashboards evolved to support distributed tracing, and SIEM systems gained prominence for log correlation and security analytics.
- The early 2020s: Observability matured into a holistic discipline focused on metrics, traces, logs, and events. Integration with AIOps enables event correlation, noise suppression, and autonomous diagnostics. Deep-dive performance analytics, synthetic monitoring, mobile app monitoring, and code-level instrumentation became common. Observability became a central enabler of DevOps and SRE practices.
What Sets Modern Observability Apart in 2025
The new era of observability is autonomous, context-aware, and business-aligned. The integration of observability with AIOps platforms has made it possible to move from passive monitoring to active orchestration of system health and user experience.
Key Advancements in 2024–2025
- Standardization with OpenTelemetry: Now adopted by over 60% of global enterprises for vendor-neutral telemetry ingestion.
- Cloud-Native Optimization: Tools natively monitor Kubernetes, serverless, and distributed systems at scale.
- Business-Centric Views: Dashboards align technical metrics with user KPIs and revenue-impacting events.
- Security Integration: Observability systems now incorporate real-time threat detection and vulnerability scanning.
- Self-Healing Infrastructure: Automation scripts and LLMs remediate common issues without manual triggers.
Conclusion
Full-stack observability is not an optional entity; it's a must-have platform for any business looking to monitor and improve its IT environment proactively. The integration of Gen AI, ML, and LLMs transforms observability from a reactive monitoring function into a proactive, intelligent system that supports:
- Better decision-making aligned with business KPIs
- Predictive incident management and self-healing
- Improved user experiences across digital touchpoints
For organizations pursuing digital transformation, this shift is critical. Observability platforms must evolve not only to track performance but also to understand business impact in real-time.
At Tech Mahindra, we are leading this evolution, partnering with global enterprises to design and implement AI-driven full-stack observability frameworks that deliver measurable value, reduce downtime, and elevate operational intelligence.
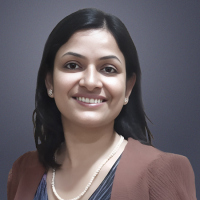
Monika is the Technical Architect for ITOM, full-stack observability implementation and support. With more than 15 years of experience across several domains, she has experience in designing, implementing, integrating, and automating solutions for multiple enterprises.
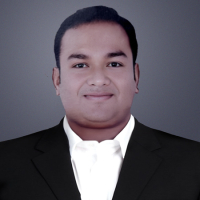
Sujeeth Sunku is the Global Delivery Head for Enterprise Service Management Solutions, responsible for implementation, Migration, consulting, and Support of all ITSM, ITOM, APM, and WLA Platforms. Sujeeth comes with more than 22 years of experience in the Enterprise Tools Domain.