Ushering in a New Era of Generative AI in Insurance
Generative AI (GenAI) technology has been in making since decades. However, it got more attention after the launch of ChatGPT by Open AI in Nov 2022. Since then, dozens of companies like Cohere, Google, Amazon, Microsoft have announced new generative AI products one after the other. Bedrock, Bard, and GPT4 are few recent additions to the generative AI domain.
GenAI is helping in automating creative activities across industries, which were earlier only dependent on human intelligence and the insurance industry is also not an exception. According to McKinsey, Insurance industry has potential of delivering value of $50 billion to $70 billion annually if the GenAI use cases are fully implemented.
This blog explains fundamentals of generative AI, relevant use cases for insurance industry and provides insights into how to operationalize GenAI to reap its benefits.
What is Generative AI and How it Works?
GenAI is a type of artificial intelligence that uses algorithms to mimic human-created content such as text, voice, images, graphics, music and more, and is designed to learn from training data that includes examples of the desired output. As a result, models can produce authentic content that share characteristics with the input data.
The power of GenAI is its large language model (LLM) trained using a huge corpus of historical data, cleansed, and curated to ensure a good quality output. The model analyzes the patterns and rules on the relationships within the data to fine tune its learning. Big players like Amazon and Google have built such LLMs by carefully tuning the inputs to the artificial neural networks and deep learning of the GenAI models.
The diagram below explains the illustrative construct of how GenAI model is trained (refer steps 1 to 3) and how it is used by end user/ consumer (boxes in red at the top).
Illustration of Generative AI Technology Landscape
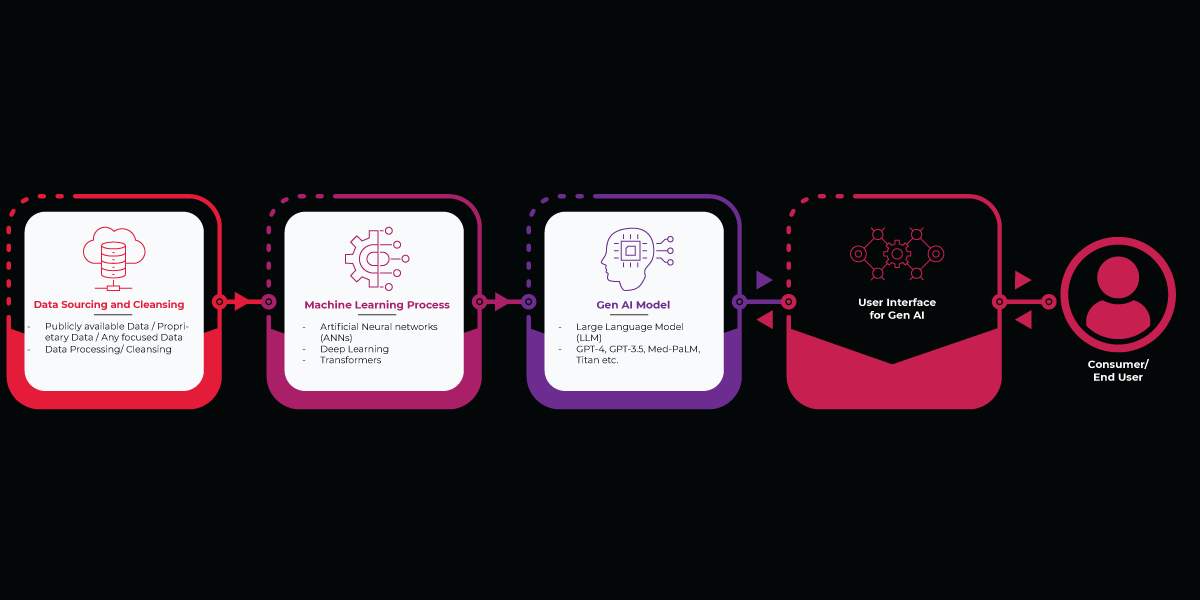
As creating an LLM from scratch is an involved task, alternate mechanisms such as fine-tuning and prompting can be adopted. The models can be operationalized through an API-like interface for safe and secure consumption.
How to Operationalize Generative AI?
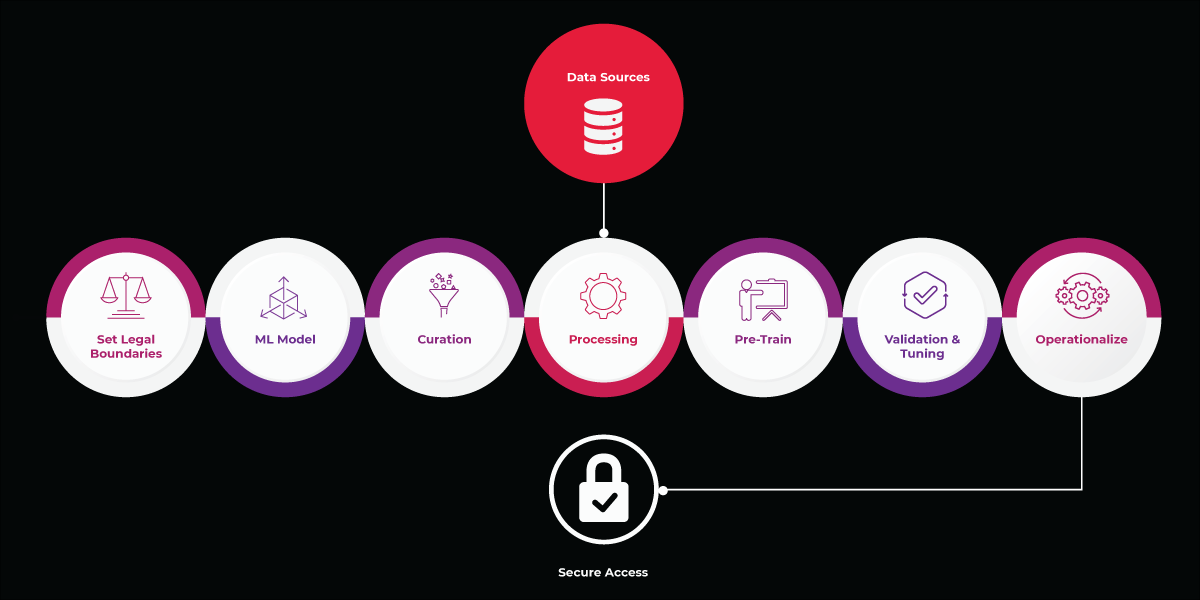
Ways to Train Gen AI Model
From Scratch: This method of creating a large language model (LLM) requires training LLMs on terabytes of curated historical data which may consume million hours of GPU and need data engineers and data scientists. This helps in tailoring LLM but is resource intensive and costly.
Fine Tuning: This method leverages an existing LLM on which data scientists apply domain specific input to the model along with training on good curated right sets of data.
Prompting: This process involves leveraging an existing domain specific LLM and training using questions and answers also known as FAQs. This model leverages the terms and language embedding of the base LLM and hence is the easiest of all the options.
Validations Before Operationalization
An integral part of operationalizing a model is validation using a canned set of golden questions, in an automated way with feedback sent to previous steps. Depending on the domain and the sensitivity of the questions, it may be necessary to add a layer of human curation. Based on the weighted risks, it may be necessary to add restrictions to the output.
How to Start Building a Model?
Model construction using one of the options above depends on the use case, data, and funds available. There are many models both enterprise level and open source to suit a variety of domains and one of which can be used to tune before operationalization. As the process involves errors enough caveats should be taken to ensure the output quality and based on the risk levels. The entire process of roll-out is iterative and evolving.
Insurance Use Cases of Generative AI
GenAI can have several potential use cases across insurance value chain. Here are a few examples:
- Customer Support: GenAI can be used to handle customer queries, provide policy information, and help in filing claims. This can help insurers reduce their workload and improve customer satisfaction.
- Personalized Policy Recommendations: GenAI can analyze customer data and generate personalized policy recommendations based on customer needs and risk profiles.
- Submission Prioritization: Underwriters processing complex submissions can leverage GenAI to prioritize and focus on the right submissions at the right time.
- Underwriting: Insurers can leverage GenAI to automate the collection and analysis of data essential for risk assessment and underwriting decisions, thereby expediting the process, and enhancing the accuracy of such decisions.
- Claims Processing: GenAI can facilitate claims adjustors to process claims effectively right from setting the claim reserves, claim assignment, claim validation and processing.
- Fraud Detection: GenAI can be used to detect fraudulent claims by analyzing claim information and identifying suspicious patterns or behaviors. This can help insurers prevent fraudulent claims and reduce their losses.
Paving the Path to a New Era of Insurance
GenAI is continuously evolving, and the insurance industry is eager to adopt it to harness the benefits. With more LLMs on the rise, insurers can choose from a wealth of options before creating their custom model. Insurers should identify appropriate use cases and weigh the risks, benefits, payoffs and how it will fit into current IT and business landscape to maximize the benefits. Insurers need to adopt incremental approach to implement GenAI as extension to the existing AI/ML use cases. Starting small, conducting pilots, identifying magnitude of risks in terms of - data privacy, errors in output, computing resources will be quintessential to realize true value.
Contributor
Arun John
Principal Consultant – Insurance
Rajan S
Competency Head – Insurance Solutions